C2I 2020
Category: Medical & Healthcare
Winner: Machine Learning for Enhanced Diabetes Care
Partners: Quin Technology Ltd with University of Bristol
A typical person with type 1 diabetes will have around 65,000 insulin injections throughout their lifetime. But despite the fact that technology is improving treatment all the time, decisions about the timing and quantity of these injections is still little more than guesswork in many cases. Quin, a new app underpinned by the winning project in our Medical & Healthcare category, is striving to change that.
The app allows users to input both insulin dosage and food consumption, as well as providing reminders for when regular insulin injections are due. Additional sensor data from smartphones such as activity, sleep and menstrual cycle can also be linked, alongside readings from CGMs (Continuous Glucose Monitors). Over time, the platform’s algorithms learn from individual inputs and outcomes and help users adjust their insulin dosage based on what has worked for them in the past.
Machine Learning for Enhanced Diabetes Care - the project from which the new app evolved - resulted from an encounter between Quin Technology CEO Cyndi Williams and Dr Aisling Ann O’Kane from the University of Bristol. Quin Technology had been founded in 2014 with the express aim of improving life for type 1 diabetes patients. Dr O’Kane, a specialist in human computer interaction (HCI) in health, had expertise in behavioural models and engagement, applying user-focused design research to empower independent patient decisions.
After sharing observations on the shortcomings of diabetes care and realising the complementary nature of their work, the two endeavoured to work together in the future. The perfect opportunity presented itself a few years later in the shape of Innovate UK’s Digital Health Technology Catalyst (DHTC) challenge, and the collaboration was born. The team explored existing data models such as the Ambulatory Glucose Profile (AGP), already widely used in diabetes treatment, adapting it to make it more useful to type 1 patients. This innovative approach fed into one of the key innovations of the Quin app – the What’s Ahead Graph that gives users predictions on their blood glucose levels for focused periods of time.
“The Ambulatory Glucose Profile concept is a form of percentile bands graph for time series which summarises a month of blood glucose data for a single person across the 24-hour day,” Cyndi Williams told The Engineer.
“This is a very generic summary and draws out very high-level patterns of blood glucose outcomes. We have similarly used the concept of percentile bands when designing the Quin graph but instead have made the display shorter - five hours, relevant to the current time of day so the user can see the patterns at the most important times - and more personalised to an individual by identifying and including relevant past blood glucose outcomes around specific user actions, such as eating food and taking insulin.”
Using machine learning, the What’s Ahead Graph becomes more personalised over time based on food and insulin recorded and previous blood glucose levels. It gives the user insight into their individual patterns and enables more informed dosage control and ultimately better patient outcomes.
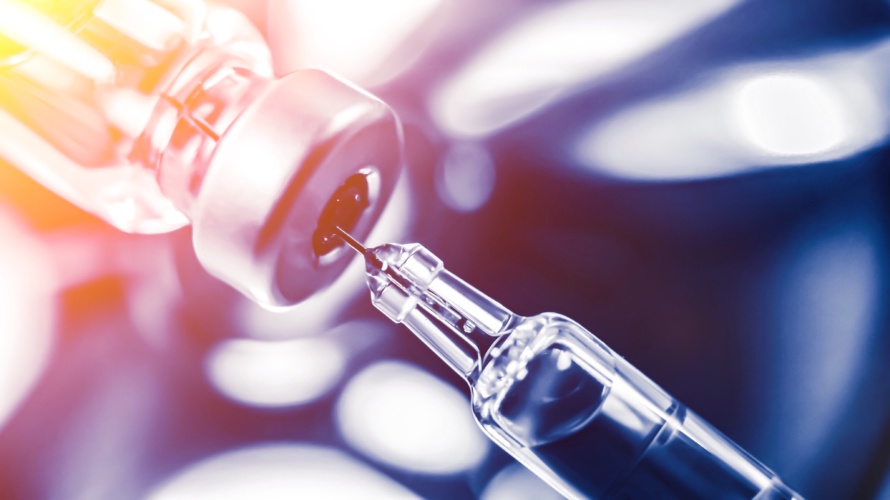
“This makes the Quin graph much more relevant to the user and incredibly useful for assisting in making real time diabetes-related decisions rather than for evaluating retrospectively with an absence of context and low-level detail with a healthcare professional,” Williams explained.
Another data innovation that feeds into the graph’s usability is the use of Kernel Density Estimate (KDE). With each personalised data set being limited in scope, there are inevitable trade-offs between precision and accuracy. Applying KDE contextualises this uncertainty and accounts to some degree for the unpredictable nature of diabetes, giving the user a more realistic and useful picture of their overall glucose levels.
“As we are working with sparse and complex data in blood glucose samples, Kernel Density Estimate has been used to create an understandable and smooth graph for the users by utilising probability density from relevant, previous blood glucose samples for the user,” said Williams.
“Without the use of KDE, the graph would display with defined percentile lines rather than a smooth flow and this would convey an unrealistic level of precision for a condition as unpredictable as diabetes. This would likely both confuse the user and be potentially unsafe but, by utilising KDE, we are able to represent useful trends to the user in an engaging and clear way.”
The collaboration between Quin and the University of Bristol has resulted in the acceleration of the app’s development into a commercially available product, which launched on Apple’s UK & Ireland App Store in September 2020. According to Williams, 84 per cent of users report having better health outcomes or quality of life, or both, since starting to use the app. Three-quarters have reported fewer instances of hyper- or hypoglycaemia, while 67 per cent have reported spending more time-in-range (TIR) of their target blood sugar, a key health metric for type 1 diabetics.
“While the data set is still small, it shows a significant opportunity to help people who spend less than 70 per cent of time in their target blood sugar range to improve by 20 per cent or more,” said Williams. “And the improvements appear to be sustained over several weeks of usage.
“By comparison, continuous glucose monitoring (CGM) — the technology taking the diabetes world by storm right now — achieved 5 per cent improvement in time-in-range in the pivotal clinical trial that unlocked widespread adoption and reimbursement for the Dexcom CGM.”
Despite the bulk of the collaboration having taken place pre-Covid, much of the work was carried out remotely using online tools such as Zoom, Slack and Google Docs, with quarterly project meetings held in person with the Innovate UK Project Monitoring team. Although Quin Technology and Bristol University were approaching the project with different perspectives and goals, ultimately the collaboration was successful due to both parties’ dedication to improving daily life for people with type 1 diabetes.
FIND OUT MORE ABOUT THIS YEAR'S MEDICAL & HEALTHCARE SHORTLIST
Quin’s 1,000 monthly active users (MAUs) are expected to grow to 10,000 by the end of 2021 as the app expands into new territories and becomes available on Android. By 2024, Williams predicts that as many as 1,000,000 MAUs could be benefitting from personalised insights into their blood glucose levels and insulin dosing. What’s more, the methodologies developed under the collaboration could potentially be applied to other chronic medical conditions where trial and error treatments are often the norm.
“Chronic conditions remain chronic because there isn’t enough scientific knowledge to treat and/or cure them,” said Williams. “This means the treatments are inexact and the people living with the condition end up using their own self-experimentation to make them work as best they can. They are effectively filling the gaps in science with their own trial and error. “Innovations on the project could also be used to capture and formalise the daily trial-and-error experiences across many individuals with the same chronic condition, and then use those insights and data sets to advance research into the condition.”
Experts speculate over cause of Iberian power outages
I´m sure politicians will be thumping tables and demanding answers - while Professor Bell, as reported above, says ´wait for detailed professional...