Known as Dense Object Nets (DON), the system uses a camera to create a visual roadmap of an object as a collection of points. These coordinates can then be referred to by the system from any angle, allowing it to identify specific objects and helping robots grab them in specific ways. Unlike other machine vision systems, DON is able to carry out tasks on objects without having seen them before or being trained on the task. The MIT team believes the technology could be applied in warehouses by logistics companies or online retailers such as Amazon.
"Many approaches to manipulation can't identify specific parts of an object across the many orientations that object may encounter," said PhD student Lucas Manuelli, who wrote a new paper about the system with lead author and fellow PhD student Pete Florence, alongside MIT professor Russ Tedrake. "For example, existing algorithms would be unable to grasp a mug by its handle, especially if the mug could be in multiple orientations, like upright, or on its side."
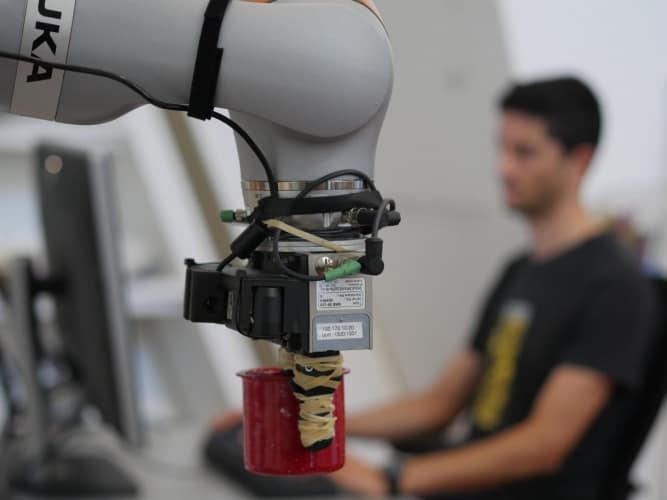
In one set of tests carried out with a soft toy, a Kuka robotic arm powered by DON was able to grasp the toy's right ear from a range of different configurations. This showed that the system has the ability to distinguish left from right on symmetrical objects. In another test on a bin of different baseball hats, DON picked out a specific target hat despite all of the hats having very similar designs and having never seen pictures of the hats in training data before.
"In factories robots often need complex part feeders to work reliably," said Manuelli. "But a system like this that can understand objects' orientations could just take a picture and be able to grasp and adjust the object accordingly."
The team will present its findings next month at the Conference on Robot Learning in Zürich, Switzerland.
Hard hat mounted air curtain adds layer of protection
Something similar was used by miners decades ago!