ROSEHIPS to drive new infrastructure standards
A project is underway to transform the way infrastructure assets including wind turbines and bridges are monitored and maintained.
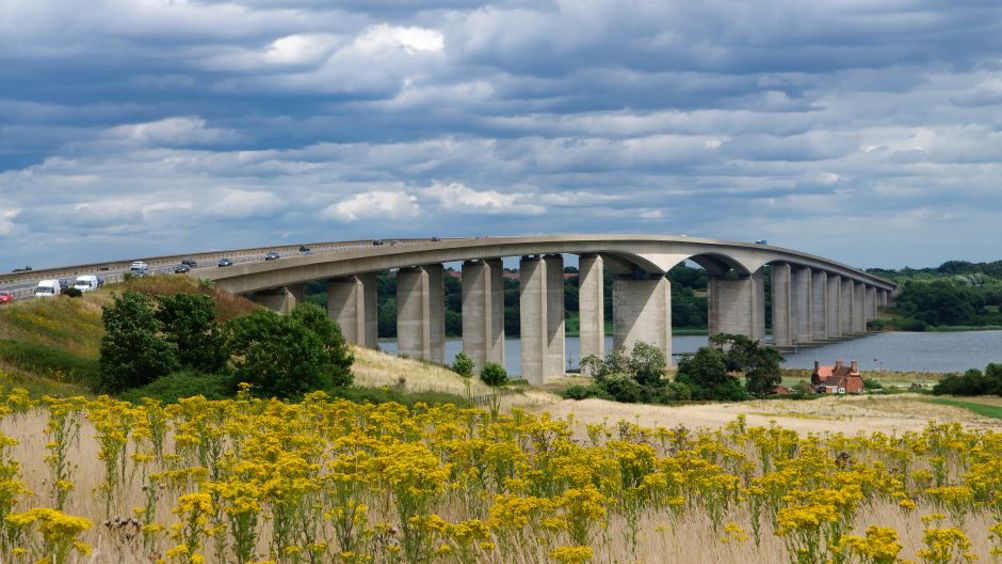
Led by Sheffield University and funded with a £7.7m EPSRC Programme Grant, the ROSEHIPS project brings together experts from academia and industry to solve the challenge of safely and economically safeguarding current and future infrastructure. ROSEHIPS runs until 2027 and involves Queen’s University Belfast, the Universities of Cambridge and Exeter, plus industry partners including Northern Ireland’s Department for Infrastructure, Translink, Arqiva, Cellnex (UK) and Siemens Gamesa.
In 2019 the cost of clearing the UK’s backlog of maintenance works was valued at £6.7bn. ROSEHIPS (Revolutionising Operational Safety and Economy for High-value Infrastructure using Population-based SHM (structural health monitoring)) aims to solve the UK’s infrastructure asset management problem through research to automate health monitoring.
Instead of expensive scheduled inspections, diagnoses can be provided economically by permanently installed sensors that continuously collect structural data and interpret it via computer algorithms that will help inform the ROSEHIPS framework.
Register now to continue reading
Thanks for visiting The Engineer. You’ve now reached your monthly limit of news stories. Register for free to unlock unlimited access to all of our news coverage, as well as premium content including opinion, in-depth features and special reports.
Benefits of registering
-
In-depth insights and coverage of key emerging trends
-
Unrestricted access to special reports throughout the year
-
Daily technology news delivered straight to your inbox
Water Sector Talent Exodus Could Cripple The Sector
Maybe if things are essential for the running of a country and we want to pay a fair price we should be running these utilities on a not for profit...