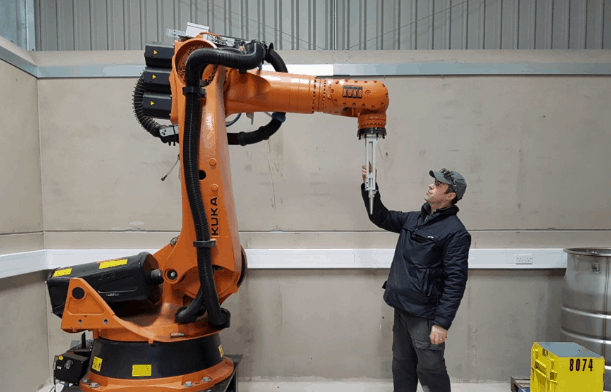
Launched in 2018, the NCNR is a consortium of eight universities led by the University of Birmingham and backed by £42m of funding. Its primary mission is to develop robotic solutions that can characterise, handle and decommission the huge amounts of waste generated by the nuclear industry over the past since the early 1950s.
Using current technology, the clean-up would take 120 years and an estimated one million human entries into contaminated zones, with a cost of approximately £234bn. According to the NCNR, developing robots capable of taking up the task is a necessity for a number of reasons.
“There’s a large amount of radioactive waste that humans can’t go near at all,” Prof Rustam Stolkin, co-director of the NCNR, told a press event at the Royal Institution. “And where we have technology that’s now becoming capable to do the complex things that human workers do, we have an ethical and moral obligation to stop using humans in those roles. We don’t send Victorian children up chimneys any more. It’s not socially acceptable.”
Where humans can deal with nuclear waste, they must be kitted out in air-fed plastic suits, usually wearing multiple layers and gloves for protection. But they must often also operate heavy tools, such as disc grinders, for dismantling the metal piping and containers that make up much of the legacy waste. It’s tiring and dangerous work, limited to just a couple of hours at a time. And for every barrel of high-level waste that is decommissioned, 11 further barrels of secondary waste – such as contaminated suits and gloves – is created. Using machines would help cut down on this secondary waste, but the sector has largely proved resistant to the adoption of robotics.
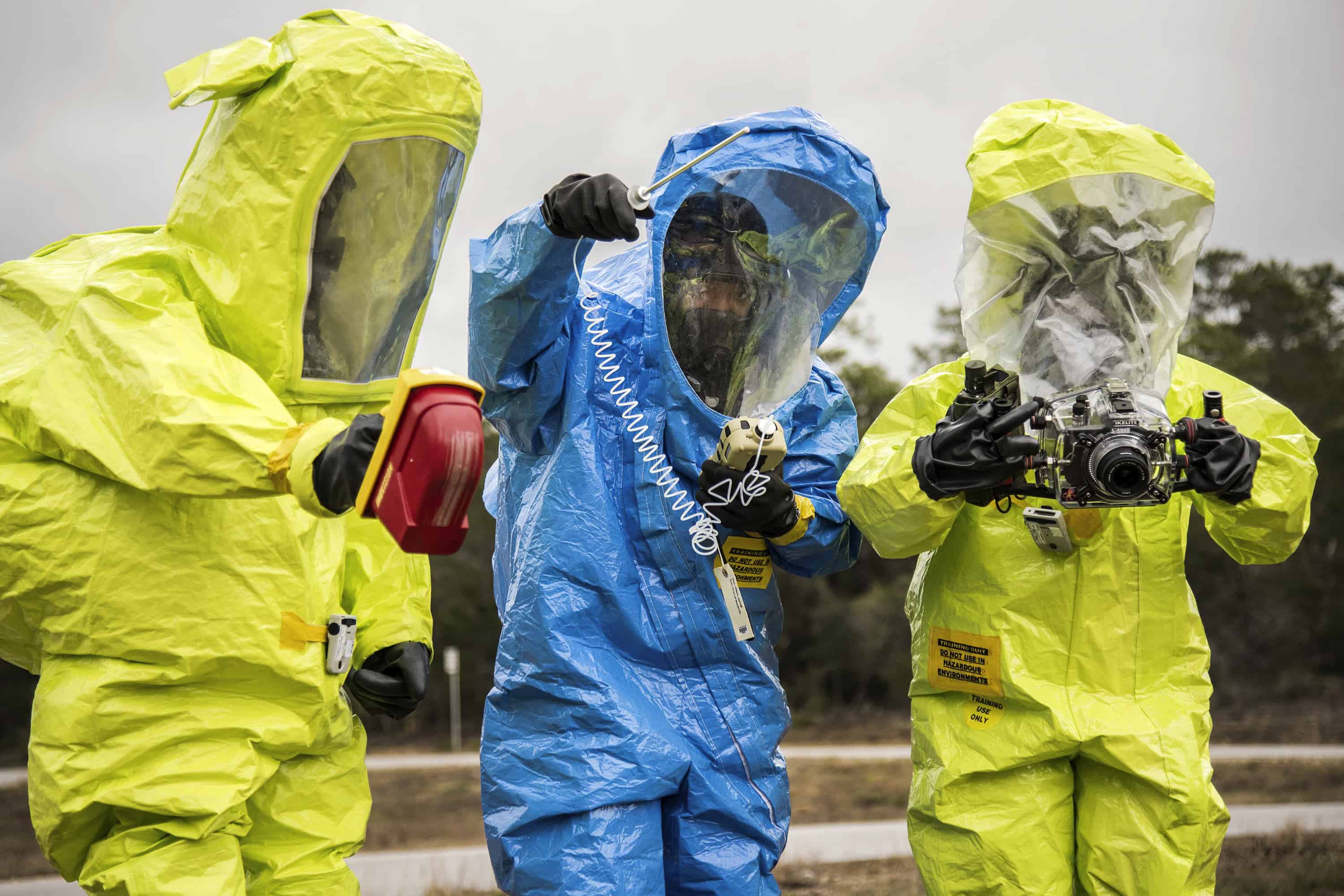
“Nuclear is a profoundly un-roboticised industry,” said Stolkin, head of Birmingham’s Extreme Robotics Lab, which is at the vanguard of NCNR’s research. “The reason that you’ve been able to have this (robotics) revolution in manufacturing, is because manufacturing is a very structured, constrained, precise environment.”
Conversely, nuclear waste is completely unstructured, and therein lies the challenge. What’s required is machine vision and AI that can characterise waste objects with no prior knowledge, enabling robots to deal with the waste autonomously. While this is a relatively simple task for a human - radiation levels notwithstanding - it is incredibly complex for a machine and represents the bleeding edge of current AI research.
“Autonomous robotic grasping is the current forefront frontier in international robotics and AI research,” Stolkin explained. “You can’t buy a robot from an industrial robot manufacturer that can do that stuff. Well, we buy the robots, but we make them behave in clever ways.”
On top of this, the robots obviously need to be resilient to high levels of radiation, as well as capable of mapping and navigating areas where no human has set foot since in 70 years. And it’s not just grasping that the robots will be required to do. NCNR has already used a robot to cut contaminated steel with a laser, the first time ever a robot has been allowed to act autonomously inside a radioactive zone.
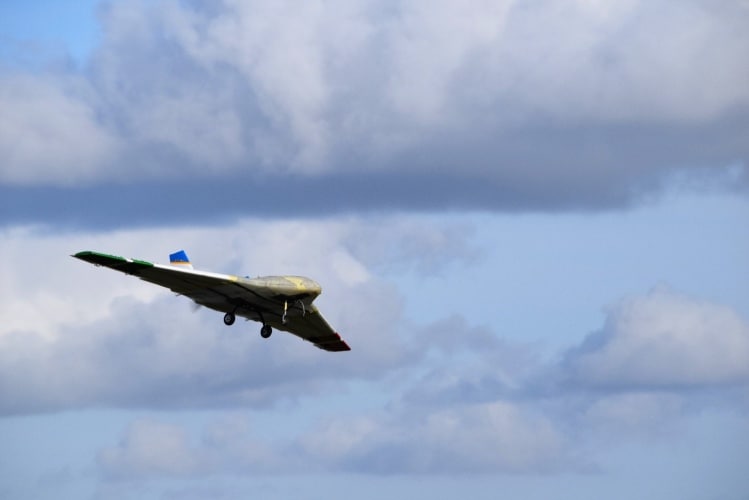
Further afield, NCNR has also been involved in operations at Chernobyl using drones to map radiation levels in the infamous ‘Red Forest’, the most highly contaminated area following the 1986 nuclear disaster at the plant. Under the lead of NCNR co-director Prof Tom Scott and working alongside Ukrainian authorities, the team successfully identified a previously unknown radiation hotspot within the forest. It’s hoped the drone mapping system, along with other NCNR advances, can be spun out to commercial applications beyond the nuclear industry.
Hard hat mounted air curtain adds layer of protection
Something similar was used by miners decades ago!