WildFusion helps robot traverse difficult terrain
Engineers have developed WildFusion, a combination of technologies that enable a robot to ‘sense’ complex outdoor environments in a similar way to humans.
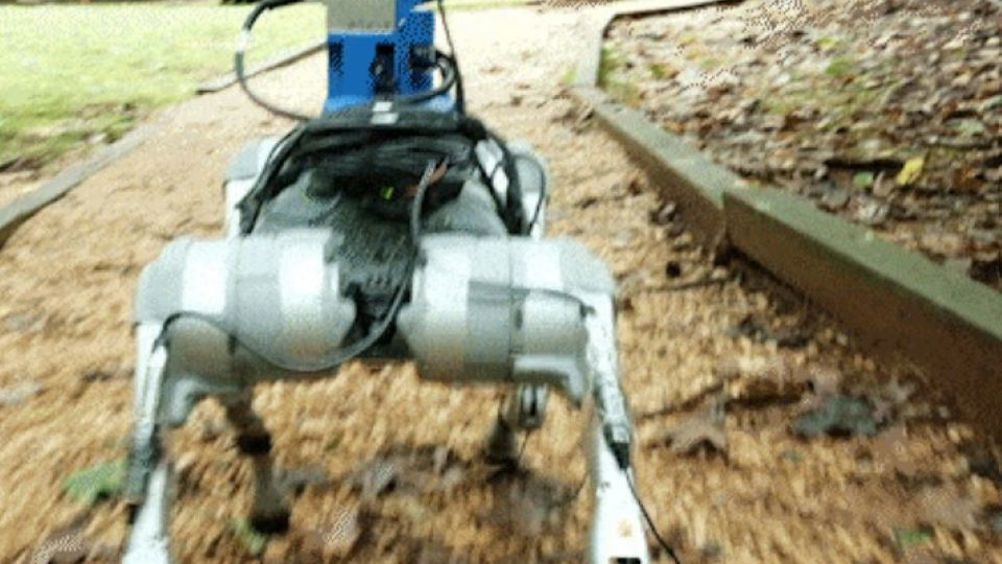
The work from Duke University, North Carolina, has been accepted to the IEEE International Conference on Robotics and Automation (ICRA 2025), which will be held May 19-23, 2025, in Atlanta, Georgia.
“WildFusion opens a new chapter in robotic navigation and 3D mapping,” said Boyuan Chen, the Dickinson Family Assistant Professor of Mechanical Engineering and Materials Science, Electrical and Computer Engineering, and Computer Science at Duke University. “It helps robots to operate more confidently in unstructured, unpredictable environments like forests, disaster zones and off-road terrain.”
"Typical robots rely heavily on vision or LiDAR alone, which often falter without clear paths or predictable landmarks," said Yanbaihui Liu, the lead student author and a second-year PhD student in Chen’s lab. “Even advanced 3D mapping methods struggle to reconstruct a continuous map when sensor data is sparse, noisy or incomplete, which is a frequent problem in unstructured outdoor environments. That’s exactly the challenge WildFusion was designed to solve.”
Register now to continue reading
Thanks for visiting The Engineer. You’ve now reached your monthly limit of news stories. Register for free to unlock unlimited access to all of our news coverage, as well as premium content including opinion, in-depth features and special reports.
Benefits of registering
-
In-depth insights and coverage of key emerging trends
-
Unrestricted access to special reports throughout the year
-
Daily technology news delivered straight to your inbox
UK Enters ‘Golden Age of Nuclear’
While I welcome the announcement of this project, I note that the original budget cost for Hinkley Point C was £18 billion and this has constantly...