The researchers from Cambridge University, in collaboration with domestic appliance company Beko, used machine learning to train the robot to account for highly subjective matters of taste. The results are reported in IEEE Robotics and Automation Letters.
Scifi Eye: Romancing the robots
Responsible robots, AI and the future of humanity
Prototype robot chefs have been built but none have been made commercially available and they lag behind their human counterparts in terms of skill.
"Cooking is a really interesting problem for roboticists, as humans can never be totally objective when it comes to food, so how do we as scientists assess whether the robot has done a good job?" said Dr Fumiya Iida from Cambridge's Department of Engineering, who led the research.
Teaching a robot to prepare and cook food presents complex problems in robot manipulation, computer vision, sensing, human-robot interaction, and the production of a consistent end-product.
Other research groups have trained robots to make biscuits, pancakes and pizza, but these robot chefs have not been optimised for the many subjective variables involved in cooking.
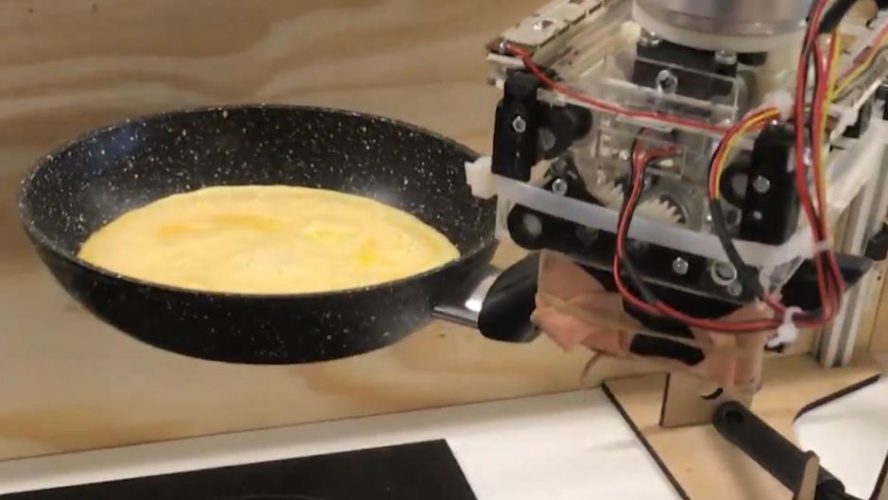
"An omelette is one of those dishes that is easy to make, but difficult to make well," Iida said in a statement. "We thought it would be an ideal test to improve the abilities of a robot chef, and optimise for taste, texture, smell and appearance."
In partnership with Beko, Iida and his colleagues trained their robot chef to prepare an omelette, from cracking the eggs through to plating the finished dish. The work was performed in Cambridge's Department of Engineering, using a test kitchen supplied by Beko and Symphony Group.
The machine learning technique developed by Iida's team is said to make use of Bayesian Inference to extract as much information as possible from the limited amount of data samples, which was necessary to avoid overwhelming the human tasters with omelettes.
"Another challenge we faced was the subjectivity of human sense of taste - humans aren't very good at giving absolute measures, and usually give relative ones when it comes to taste," Iida said. "So we needed to tweak the machine learning algorithm - the so-called batch algorithm - so that human tasters could give information based on comparative evaluations, rather than sequential ones."
"The omelettes in general tasted great - much better than expected!" said Iida.
According to Cambridge University, the results show that machine learning can be used to obtain quantifiable improvements in food optimisation. Additionally, such an approach can be easily extended to multiple robotic chefs. Further studies have to be conducted to investigate other optimisation techniques and their viability.
Glasgow trial explores AR cues for autonomous road safety
They've ploughed into a few vulnerable road users in the past. Making that less likely will make it spectacularly easy to stop the traffic for...