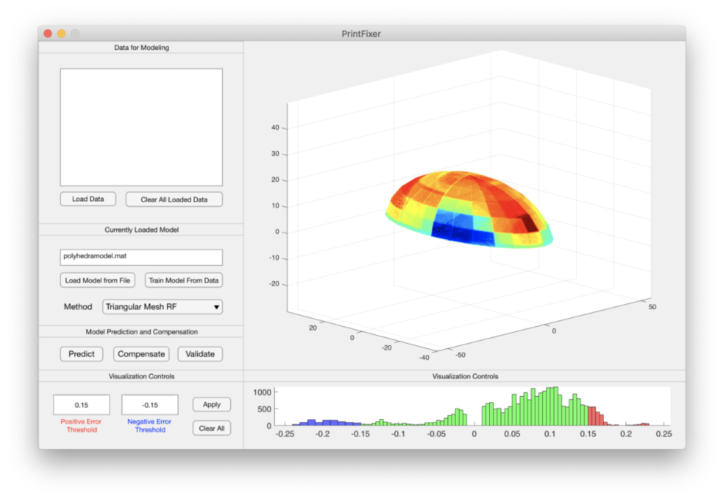
The process, which predicts shape deviations for all types of 3D printing, has been dubbed ‘convolution modelling of 3D printing’ and is described in IEEE Transactions on Automation Science and Engineering.
How collaboration is driving advances in additive manufacturing
Additive manufacturing can reduce industry’s environmental impact
"What we have demonstrated so far is that in printed examples the accuracy can improve around 50 per cent or more," said team leader Qiang Huang, associate professor of industrial and systems engineering, chemical engineering and materials science. "In cases where we are producing a 3D object similar to the training cases, overall accuracy improvement can be as high as 90 per cent."
"It can actually take industry eight iterative builds to get one part correct, for various reasons, and this is for metal, so it's very expensive," Huang said.
According to the team, every 3D printed object contains some slight deviation from the design, whether this is due to printed material expanding or contracting when printed, or due to the way the printer behaves.
PrintFixer uses data from previous 3D print jobs to train its AI to predict where the shape distortion will happen, in order to fix print errors before they occur.
Huang said that the research team had aimed to create a model that produced accurate results using the minimum amount of 3D printing source data.
"From just five to eight selected objects, we can learn a lot of useful information," Huang said in a statement. "We can leverage small amounts of data to make predictions for a wide range of objects."
The team has reportedly trained the model to work with the same accuracy across a variety of applications and materials - from metals for aerospace manufacturing, to thermal plastics for commercial use. The researchers are also working with a dental clinic in Australia on the 3D printing of dental models.
Nathan Decker, a PhD student who led the software development, said that users could opt to print with a different, higher-quality printer and use the software to predict whether that would provide a better result.
"But if you don't want to change the printer, we also have incorporated functionality into the software package allowing the user to compensate for the errors and change the object's shape - to take the parts that are too small and increase their size, while decreasing the parts that are too big," Decker said. "And then, when they print, they should print with the correct size the first time."
The team's objective is for the software tool to be available to everyone, from large scale commercial manufacturers to 3D printing hobbyists. Users from around the world will also be able to contribute to improving the software AI through sharing of print output data.
Poll: Should the UK’s railways be renationalised?
I think that a network inclusive of the vehicles on it would make sense. However it remains to be seen if there is any plan for it to be for the...